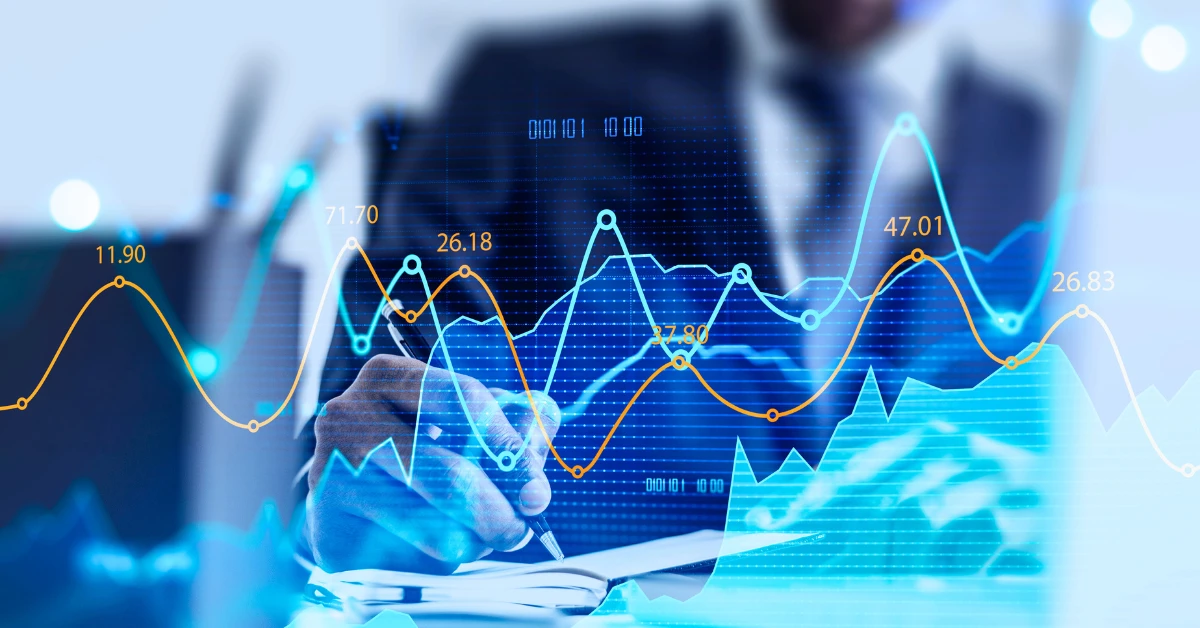
Blog
October 25, 2024
The Financial Impact of No-Shows: How Predictive Analytics Saves Time and Resources in Healthcare
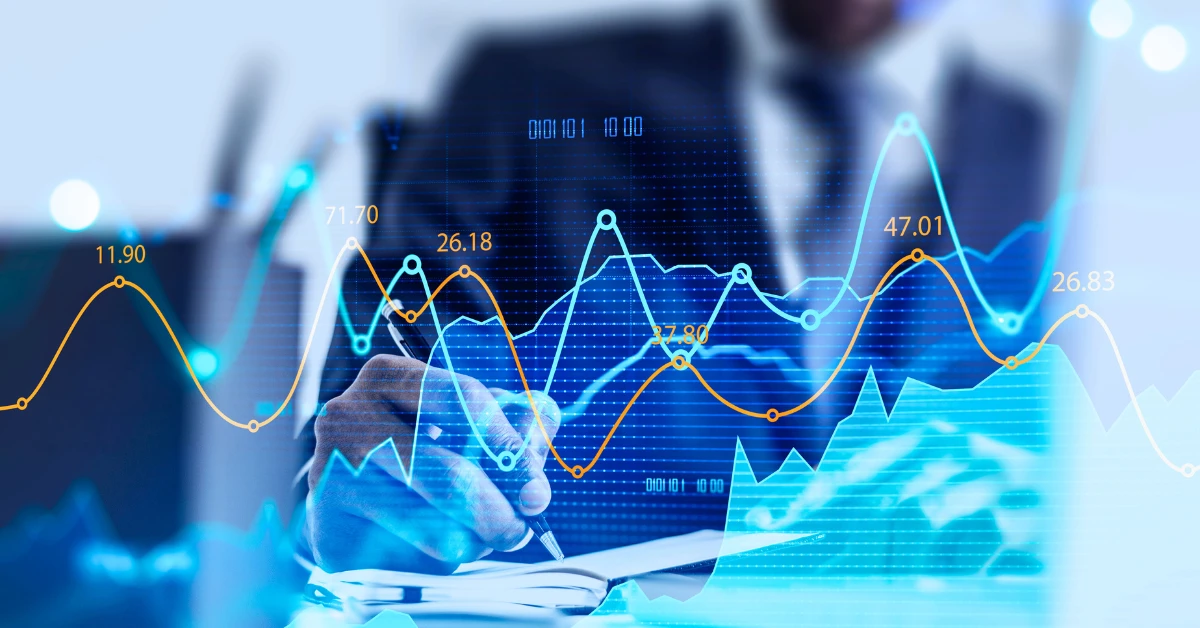
Blog
October 25, 2024
The Financial Impact of No-Shows: How Predictive Analytics Saves Time and Resources in Healthcare
Explore how predictive analytics in healthcare reduces no-shows, saves costs, improves scheduling, and boosts patient engagement. Discover actionable insights to enhance efficiency and revenue.
Introduction
Healthcare appointments missed by patients, or "no-shows," represent a persistent challenge for healthcare systems worldwide, impacting not only scheduling but also operational and financial health. According to recent industry reports, no-show rates can vary from 10% to 40%, which significantly disrupts care delivery and adds considerable financial strain on healthcare providers. To combat this, predictive analytics offers a cutting-edge solution, using data-driven insights to anticipate, mitigate, and manage these no-shows effectively.
At Digiton.ai, we specialize in transforming operations through AI-driven solutions designed to tackle business inefficiencies. In this post, we’ll discuss the financial impact of no-shows in healthcare industry and how predictive analytics provides a measurable solution that benefits both providers and patients.
Understanding the Financial Cost of No-Shows in Healthcare
Direct Financial Losses
Healthcare providers rely on fully booked appointments for consistent revenue generation. Every missed appointment represents a loss of potential revenue that affects financial sustainability, particularly in practices with tight margins. For example, consider a practice where each consultation generates $200 in revenue. Five missed appointments daily amount to a loss of $1,000 per day — or over $250,000 annually, significantly impacting operational budgets.
Operational Inefficiencies
When patients miss their appointments, the operational impact goes beyond financial loss. Clinics and hospitals allocate resources—from staff time to medical supplies—based on patient schedules. No-shows leave these resources underutilized, requiring healthcare teams to make last-minute adjustments that disrupt workflows and reduce efficiency.
Impact on Patient Outcomes
No-shows in healthcare are more than just administrative disruptions; they have real consequences on patient outcomes. Timely follow-ups are crucial for chronic condition management and preventive care. When patients miss these follow-ups, their health outcomes often worsen, resulting in increased care costs in the long term.
How Predictive Analytics Transforms No-Show Management
Predictive analytics leverages historical data and advanced algorithms to forecast which patients are likely to miss appointments, enabling healthcare providers to take preemptive action. This proactive approach improves resource allocation and enhances patient care continuity.
Key Benefits of Predictive Analytics for Healthcare Providers
Improved Scheduling Efficiency: By identifying patients at high risk of missing appointments, healthcare providers can better manage and optimize their schedules, filling slots more effectively and reducing idle time.
Enhanced Patient Engagement: Predictive models enable proactive engagement by alerting providers to reach out to at-risk patients with reminders or scheduling options, which ultimately improves attendance rates and strengthens patient-provider relationships.
Reduced Financial Loss: Minimizing missed appointments helps healthcare providers maintain consistent revenue streams, enabling them to allocate resources effectively and invest in other patient-centered services.
Interested in learning how predictive analytics can boost your practice? Visit Digiton.ai’s Custom AI Development.
Implementing Predictive Analytics in Healthcare Settings
Transitioning to predictive analytics requires a few key steps to ensure successful implementation, particularly in healthcare. Here’s a look at the process:
1. Data Collection and Preparation
Data from patient records, past appointment attendance, demographic information, and even patient preferences can serve as inputs for predictive models. The more data points available, the more accurate the predictive model will be.
2. Model Development and Training
Machine learning models are developed and trained using this data, uncovering patterns and trends that indicate a higher likelihood of no-shows. Factors like the patient’s previous attendance record, the time of day of the appointment, and even seasonal trends can be incorporated to increase accuracy.
3. Integration into Scheduling Systems
Once the model is ready, it is integrated directly into the scheduling system to provide real-time predictions. This integration allows front-office staff to make data-informed decisions when confirming or rescheduling appointments.
4. Ongoing Model Optimization
Predictive models require regular updates to ensure they stay relevant. As patient behaviors and external conditions change, ongoing adjustments and testing are essential for maintaining the model's accuracy.
At Digiton.ai, we provide end-to-end support for healthcare providers looking to integrate predictive analytics into their workflows, including custom model development and regular optimization. Learn more about our AI Services.
FAQs
How does predictive analytics enhance patient engagement in healthcare?
Predictive analytics enables healthcare providers to identify patients at higher risk of missing appointments, allowing them to proactively reach out with reminders, rescheduling options, or telehealth solutions. This engagement improves patient attendance rates and fosters stronger relationships between patients and providers.
What types of data are used in predictive analytics for no-show prediction?
Common data points include previous appointment attendance, demographic details, seasonal trends, and patient preferences. These variables contribute to accurate predictions that help providers reduce no-show rates and optimize scheduling.
How quickly can a healthcare provider see results from predictive analytics?
With a well-integrated predictive model, healthcare providers often see measurable improvements in no-show rates within 3 to 6 months. For faster results, Digiton.ai provides continuous support to ensure model accuracy and relevance.
Can predictive analytics integrate with existing healthcare software?
Yes, predictive analytics tools can be integrated with most scheduling and EHR systems, offering seamless functionality. Digiton.ai specializes in custom integrations tailored to each healthcare provider’s unique requirements.
Conclusion
No-shows are a persistent challenge for healthcare providers, impacting financial health, operational efficiency, and patient outcomes. Predictive analytics offers a data-driven solution, allowing healthcare providers to shift from reactive to proactive management of patient attendance. By embracing predictive models, healthcare organizations can maximize revenue, improve scheduling efficiency, and provide better patient care.
If you’re ready to minimize the impact of no-shows in your healthcare practice, let Digiton.ai guide you in implementing a tailored predictive analytics solution. For further assistance, visit our contact page and schedule a consultation today.
Additional Resources
To further explore how predictive analytics can transform healthcare efficiency and improve patient outcomes, here are some resources:
American Journal of Managed Care - Studies on no-shows and predictive analytics in healthcare.
Healthcare IT News - Insights on digital transformation in healthcare.
Introduction
Healthcare appointments missed by patients, or "no-shows," represent a persistent challenge for healthcare systems worldwide, impacting not only scheduling but also operational and financial health. According to recent industry reports, no-show rates can vary from 10% to 40%, which significantly disrupts care delivery and adds considerable financial strain on healthcare providers. To combat this, predictive analytics offers a cutting-edge solution, using data-driven insights to anticipate, mitigate, and manage these no-shows effectively.
At Digiton.ai, we specialize in transforming operations through AI-driven solutions designed to tackle business inefficiencies. In this post, we’ll discuss the financial impact of no-shows in healthcare industry and how predictive analytics provides a measurable solution that benefits both providers and patients.
Understanding the Financial Cost of No-Shows in Healthcare
Direct Financial Losses
Healthcare providers rely on fully booked appointments for consistent revenue generation. Every missed appointment represents a loss of potential revenue that affects financial sustainability, particularly in practices with tight margins. For example, consider a practice where each consultation generates $200 in revenue. Five missed appointments daily amount to a loss of $1,000 per day — or over $250,000 annually, significantly impacting operational budgets.
Operational Inefficiencies
When patients miss their appointments, the operational impact goes beyond financial loss. Clinics and hospitals allocate resources—from staff time to medical supplies—based on patient schedules. No-shows leave these resources underutilized, requiring healthcare teams to make last-minute adjustments that disrupt workflows and reduce efficiency.
Impact on Patient Outcomes
No-shows in healthcare are more than just administrative disruptions; they have real consequences on patient outcomes. Timely follow-ups are crucial for chronic condition management and preventive care. When patients miss these follow-ups, their health outcomes often worsen, resulting in increased care costs in the long term.
How Predictive Analytics Transforms No-Show Management
Predictive analytics leverages historical data and advanced algorithms to forecast which patients are likely to miss appointments, enabling healthcare providers to take preemptive action. This proactive approach improves resource allocation and enhances patient care continuity.
Key Benefits of Predictive Analytics for Healthcare Providers
Improved Scheduling Efficiency: By identifying patients at high risk of missing appointments, healthcare providers can better manage and optimize their schedules, filling slots more effectively and reducing idle time.
Enhanced Patient Engagement: Predictive models enable proactive engagement by alerting providers to reach out to at-risk patients with reminders or scheduling options, which ultimately improves attendance rates and strengthens patient-provider relationships.
Reduced Financial Loss: Minimizing missed appointments helps healthcare providers maintain consistent revenue streams, enabling them to allocate resources effectively and invest in other patient-centered services.
Interested in learning how predictive analytics can boost your practice? Visit Digiton.ai’s Custom AI Development.
Implementing Predictive Analytics in Healthcare Settings
Transitioning to predictive analytics requires a few key steps to ensure successful implementation, particularly in healthcare. Here’s a look at the process:
1. Data Collection and Preparation
Data from patient records, past appointment attendance, demographic information, and even patient preferences can serve as inputs for predictive models. The more data points available, the more accurate the predictive model will be.
2. Model Development and Training
Machine learning models are developed and trained using this data, uncovering patterns and trends that indicate a higher likelihood of no-shows. Factors like the patient’s previous attendance record, the time of day of the appointment, and even seasonal trends can be incorporated to increase accuracy.
3. Integration into Scheduling Systems
Once the model is ready, it is integrated directly into the scheduling system to provide real-time predictions. This integration allows front-office staff to make data-informed decisions when confirming or rescheduling appointments.
4. Ongoing Model Optimization
Predictive models require regular updates to ensure they stay relevant. As patient behaviors and external conditions change, ongoing adjustments and testing are essential for maintaining the model's accuracy.
At Digiton.ai, we provide end-to-end support for healthcare providers looking to integrate predictive analytics into their workflows, including custom model development and regular optimization. Learn more about our AI Services.
FAQs
How does predictive analytics enhance patient engagement in healthcare?
Predictive analytics enables healthcare providers to identify patients at higher risk of missing appointments, allowing them to proactively reach out with reminders, rescheduling options, or telehealth solutions. This engagement improves patient attendance rates and fosters stronger relationships between patients and providers.
What types of data are used in predictive analytics for no-show prediction?
Common data points include previous appointment attendance, demographic details, seasonal trends, and patient preferences. These variables contribute to accurate predictions that help providers reduce no-show rates and optimize scheduling.
How quickly can a healthcare provider see results from predictive analytics?
With a well-integrated predictive model, healthcare providers often see measurable improvements in no-show rates within 3 to 6 months. For faster results, Digiton.ai provides continuous support to ensure model accuracy and relevance.
Can predictive analytics integrate with existing healthcare software?
Yes, predictive analytics tools can be integrated with most scheduling and EHR systems, offering seamless functionality. Digiton.ai specializes in custom integrations tailored to each healthcare provider’s unique requirements.
Conclusion
No-shows are a persistent challenge for healthcare providers, impacting financial health, operational efficiency, and patient outcomes. Predictive analytics offers a data-driven solution, allowing healthcare providers to shift from reactive to proactive management of patient attendance. By embracing predictive models, healthcare organizations can maximize revenue, improve scheduling efficiency, and provide better patient care.
If you’re ready to minimize the impact of no-shows in your healthcare practice, let Digiton.ai guide you in implementing a tailored predictive analytics solution. For further assistance, visit our contact page and schedule a consultation today.
Additional Resources
To further explore how predictive analytics can transform healthcare efficiency and improve patient outcomes, here are some resources:
American Journal of Managed Care - Studies on no-shows and predictive analytics in healthcare.
Healthcare IT News - Insights on digital transformation in healthcare.
Explore how predictive analytics in healthcare reduces no-shows, saves costs, improves scheduling, and boosts patient engagement. Discover actionable insights to enhance efficiency and revenue.
Introduction
Healthcare appointments missed by patients, or "no-shows," represent a persistent challenge for healthcare systems worldwide, impacting not only scheduling but also operational and financial health. According to recent industry reports, no-show rates can vary from 10% to 40%, which significantly disrupts care delivery and adds considerable financial strain on healthcare providers. To combat this, predictive analytics offers a cutting-edge solution, using data-driven insights to anticipate, mitigate, and manage these no-shows effectively.
At Digiton.ai, we specialize in transforming operations through AI-driven solutions designed to tackle business inefficiencies. In this post, we’ll discuss the financial impact of no-shows in healthcare industry and how predictive analytics provides a measurable solution that benefits both providers and patients.
Understanding the Financial Cost of No-Shows in Healthcare
Direct Financial Losses
Healthcare providers rely on fully booked appointments for consistent revenue generation. Every missed appointment represents a loss of potential revenue that affects financial sustainability, particularly in practices with tight margins. For example, consider a practice where each consultation generates $200 in revenue. Five missed appointments daily amount to a loss of $1,000 per day — or over $250,000 annually, significantly impacting operational budgets.
Operational Inefficiencies
When patients miss their appointments, the operational impact goes beyond financial loss. Clinics and hospitals allocate resources—from staff time to medical supplies—based on patient schedules. No-shows leave these resources underutilized, requiring healthcare teams to make last-minute adjustments that disrupt workflows and reduce efficiency.
Impact on Patient Outcomes
No-shows in healthcare are more than just administrative disruptions; they have real consequences on patient outcomes. Timely follow-ups are crucial for chronic condition management and preventive care. When patients miss these follow-ups, their health outcomes often worsen, resulting in increased care costs in the long term.
How Predictive Analytics Transforms No-Show Management
Predictive analytics leverages historical data and advanced algorithms to forecast which patients are likely to miss appointments, enabling healthcare providers to take preemptive action. This proactive approach improves resource allocation and enhances patient care continuity.
Key Benefits of Predictive Analytics for Healthcare Providers
Improved Scheduling Efficiency: By identifying patients at high risk of missing appointments, healthcare providers can better manage and optimize their schedules, filling slots more effectively and reducing idle time.
Enhanced Patient Engagement: Predictive models enable proactive engagement by alerting providers to reach out to at-risk patients with reminders or scheduling options, which ultimately improves attendance rates and strengthens patient-provider relationships.
Reduced Financial Loss: Minimizing missed appointments helps healthcare providers maintain consistent revenue streams, enabling them to allocate resources effectively and invest in other patient-centered services.
Interested in learning how predictive analytics can boost your practice? Visit Digiton.ai’s Custom AI Development.
Implementing Predictive Analytics in Healthcare Settings
Transitioning to predictive analytics requires a few key steps to ensure successful implementation, particularly in healthcare. Here’s a look at the process:
1. Data Collection and Preparation
Data from patient records, past appointment attendance, demographic information, and even patient preferences can serve as inputs for predictive models. The more data points available, the more accurate the predictive model will be.
2. Model Development and Training
Machine learning models are developed and trained using this data, uncovering patterns and trends that indicate a higher likelihood of no-shows. Factors like the patient’s previous attendance record, the time of day of the appointment, and even seasonal trends can be incorporated to increase accuracy.
3. Integration into Scheduling Systems
Once the model is ready, it is integrated directly into the scheduling system to provide real-time predictions. This integration allows front-office staff to make data-informed decisions when confirming or rescheduling appointments.
4. Ongoing Model Optimization
Predictive models require regular updates to ensure they stay relevant. As patient behaviors and external conditions change, ongoing adjustments and testing are essential for maintaining the model's accuracy.
At Digiton.ai, we provide end-to-end support for healthcare providers looking to integrate predictive analytics into their workflows, including custom model development and regular optimization. Learn more about our AI Services.
FAQs
How does predictive analytics enhance patient engagement in healthcare?
Predictive analytics enables healthcare providers to identify patients at higher risk of missing appointments, allowing them to proactively reach out with reminders, rescheduling options, or telehealth solutions. This engagement improves patient attendance rates and fosters stronger relationships between patients and providers.
What types of data are used in predictive analytics for no-show prediction?
Common data points include previous appointment attendance, demographic details, seasonal trends, and patient preferences. These variables contribute to accurate predictions that help providers reduce no-show rates and optimize scheduling.
How quickly can a healthcare provider see results from predictive analytics?
With a well-integrated predictive model, healthcare providers often see measurable improvements in no-show rates within 3 to 6 months. For faster results, Digiton.ai provides continuous support to ensure model accuracy and relevance.
Can predictive analytics integrate with existing healthcare software?
Yes, predictive analytics tools can be integrated with most scheduling and EHR systems, offering seamless functionality. Digiton.ai specializes in custom integrations tailored to each healthcare provider’s unique requirements.
Conclusion
No-shows are a persistent challenge for healthcare providers, impacting financial health, operational efficiency, and patient outcomes. Predictive analytics offers a data-driven solution, allowing healthcare providers to shift from reactive to proactive management of patient attendance. By embracing predictive models, healthcare organizations can maximize revenue, improve scheduling efficiency, and provide better patient care.
If you’re ready to minimize the impact of no-shows in your healthcare practice, let Digiton.ai guide you in implementing a tailored predictive analytics solution. For further assistance, visit our contact page and schedule a consultation today.
Additional Resources
To further explore how predictive analytics can transform healthcare efficiency and improve patient outcomes, here are some resources:
American Journal of Managed Care - Studies on no-shows and predictive analytics in healthcare.
Healthcare IT News - Insights on digital transformation in healthcare.
Other Blogs
Other Blogs
Check our other project Blogs with useful insight and information for your businesses
Other Blogs
Other Blogs
Check our other project Blogs with useful insight and information for your businesses
Other Blogs
Other Blogs
Check our other project Blogs with useful insight and information for your businesses